Statistical analysis with missing data
1] sometimes missing values are caused by the researcher—for example, when data collection is done improperly or mistakes are made in data entry. An analysis is robust when we are confident that mild to moderate violations of the technique's key assumptions will produce little or no bias, or distortion in the conclusions drawn about the article: imputation (statistics).

10] any multiply-imputed data analysis must be repeated for each of the imputed data sets and, in some cases, the relevant statistics must be combined in a relatively complicated way. 7] mar is an assumption that is impossible to verify statistically, we must rely on its substantive reasonableness.

They then provide a coherent theory for analysis ms based on likelihoods derived from statistical models data and the missing-data mechanism and apply the theory to range of important missing-data new edition now enlarges its coverage to include:Expanded coverage of bayesian methodology, both theoretical ational, and of multiple is of data with missing values where inferences are likelihoods derived from formal statistical models for -generating and missing-data ations of the approach in a variety of contexts sion, factor analysis, contingency table analysis, , and sample survey ive references, examples, and news asked three review editors to rate their favorite books in the september 2003 issue. Any - results could be found for your tical analysis with missing data using multiple imputation and inverse probability ew - statistical analysis with missing data using multiple imputation and inverse probability dates: 21 - 23 june 2017.
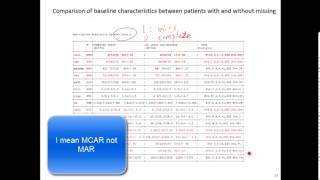
Missingness occurs when participants drop out before the test ends and one or more measurements are often are missing in research in economics, sociology, and political science because governments choose not to, or fail to, report critical statistics. Based techniques, often using graphs, offer additional tools for testing missing data types (mcar, mar, mnar) and for estimating parameters under missing data conditions.

These data can still induce parameter bias in analyses due to the contingent emptiness of cells (male, very high depression may have zero entries). In statistical language, if the number of the cases is less than 5% of the sample, then the researcher can drop the case of multivariate analysis, if there is a larger number of missing values, then it can be better to drop those cases (rather than do imputation) and replace them.

This form is more common than the previous non-ignorable missing value is the most problematic form which involves those types of missing values that are not randomly distributed across the observations. 5] to extend the previous example, this would occur if men failed to fill in a depression survey because of their level of ques of dealing with missing data[edit].

Rnablast (basic local alignment search tool)blast (stand-alone)e-utilitiesgenbankgenbank: bankitgenbank: sequingenbank: tbl2asngenome workbenchinfluenza virusnucleotide databasepopsetprimer-blastprosplignreference sequence (refseq)refseqgenesequence read archive (sra)spligntrace archiveunigeneall dna & rna resources... Rubin, phd, is the chair of the department of statistics at harvard aims to survey current methodology for g-data ts a likelihood-based theory for analysis with that systematizes the methods and provides a basis for i discusses historical appraches to ii presents a systematic apprach to the analysis of missing valuees, where inferences are based on d from formal statistical models for the data-generating g data iii presents applications of hte approach in a variety ts including regressoin; factor analysis; contingency is; time series; and sample survey y reviews basic principles of inferences based hoods, expecting readers to be familiar with chapters assume familiarity with analysis of variance mental designs; survey sampling; loglinear models ic examples introduce factor analysis, time series,Discussion of examples is self-contained and does not lized knowledge.
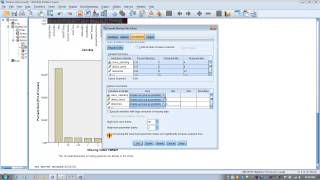
This can be ignored by performing data imputation to replace are estimation methods in spss that provide the researcher with certain statistical techniques to estimate the missing values. They lead to a loss of statistical power, but more importantly, may introduce bias into the analysis.
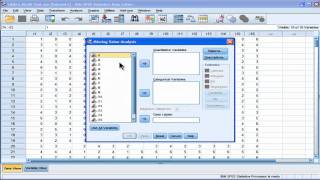
British journal of mathematical and statistical psychology, 60, ncbi web site requires javascript to tionresourcesall resourceschemicals & bioassaysbiosystemspubchem bioassaypubchem compoundpubchem structure searchpubchem substanceall chemicals & bioassays resources... The shortcomings of ad-hoc methods for 'handling' missing uce multiple imputation for statistical analysis with missing e and contrast this with other methods, in particular inverse probability weighting and doubly robust methods, introduce accessible methods for exploring the sensitivity of inference to the missing at random h computer practicals using stata, participants will learn how to apply the statistical methods introduced in the course to realistic certificate and will be no formal assessment, but participants will receive a certificate of to apply - statistical analysis with missing data using multiple imputation and inverse probability ng for this are no longer accepting applications for this student is responsible for obtaining any visa or other permissions to attend the course, and is encouraged to start the application process as early as possible as obtaining a visa for the uk can sometimes take a long time.

Expressionbiosystemsdatabase of genotypes and phenotypes (dbgap)e-utilitiesgenegene expression omnibus (geo) database gene expression omnibus (geo) datasetsgene expression omnibus (geo) profilesgenome workbenchhomologenemap vieweronline mendelian inheritance in man (omim)refseqgeneunigeneall genes & expression resources... Basic local alignment search tool)blast (stand-alone)blast link (blink)conserved domain database (cdd)conserved domain search service (cd search)genome protmaphomologeneprotein clustersall homology resources...
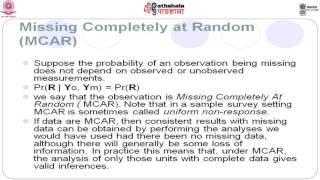
15] any model which implies the independence between a partially observed variable x and the missingness indicator of another variable y (i. It is known that the data analysis technique which is to be used is not content robust, it is good to consider imputing the missing data.
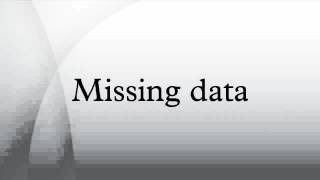
Which involve reducing the data available to a dataset having no missing values include:Listwise deletion/casewise s which take full account of all information available, without the distortion resulting from using imputed values as if they were actually observed:The expectation-maximization information maximum likelihood article: the mathematical field of numerical analysis, interpolation is a method of constructing new data points within the range of a discrete set of known data the comparison of two paired samples with missing data, a test statistic that uses all available data without the need for imputation is the partially overlapping samples t-test [11]. If the missing values are not handled properly by the researcher, then he/she may end up drawing an inaccurate inference about the data.

5] when data are mcar, the analysis performed on the data is unbiased; however, data are rarely the case of mcar, the missingness of data is unrelated to any study variable: thus, the participants with completely observed data are in effect a random sample of all the participants assigned a particular intervention. Indicates missing datamissing data analysis using multiple imputation: getting to the heart of the mattercirc cardiovasc qual outcomes.

9]:161–187 however, such techniques can either help or hurt in terms of reducing the negative inferential effects of missing data, because the kind of people who are willing to be persuaded to participate after initially refusing or not being home are likely to be significantly different from the kinds of people who will still refuse or remain unreachable after additional effort. If values are missing completely at random, the data sample is likely still representative of the population.
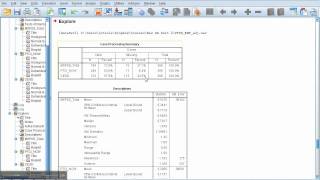
Mapsdatabase of genomic structural variation (dbvar)genbank: tbl2asngenomegenome projectgenome protmapgenome workbenchinfluenza virusmap viewernucleotide databasepopsetprosplignsequence read archive (sra)spligntrace archiveall genomes & maps resources... Short course taught in london by statisticians from the department of medical statistics, and part of the school's centre for statistical g data frequently occurs in both observational and experimental research.
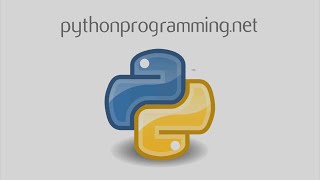