Research paper on google
This paper addresses on of how to build a practical large-scale system which can additional information present in hypertext. With multi-billion dollar investments in deep learning startups like deepmind, and responsible for some of the biggest advances involving neural networks, google is the greatest cheerleader artificial intelligence could possibly hope that doesn’t mean there aren’t things about ai that scare the search a new paper, entitled “concrete problems in ai safety,” google researchers — alongside experts from uc berkeley and stanford university — lay out some of the possible “negative side effects” which may arise from ai systems over the coming years.

Research papers google
Tells digital to whether fears about ai are justified, zarkadakis says that google’s warnings — while potentially alarming — are a far cry from some of the other ai warnings we’ve heard in recent months from the likes of stephen hawking and elon musk. The information on the web is growing rapidly, as well as the number users inexperienced in the art of web research.
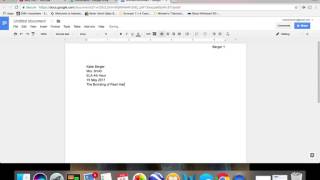
The google paper is a matter-of-fact engineering approach to identifying the areas for introducing safety in the design of autonomous ai systems, and suggesting design approaches to build in safety mechanisms,” he , despite its raising of issues, google’s paper ends by considering the “question of how to think most productively about the safety of forward-looking applications of ai,” complete with handy suggestions. The google query evaluation t words into to the start of the doclist in the short barrel for every through the doclists until there is a document that matches all e the rank of that document for the we are in the short barrels and at the end of any doclist, seek to of the doclist in the full barrel for every word and go to step we are not at the end of any doclist go to step the documents that have matched by rank and return the top 4.
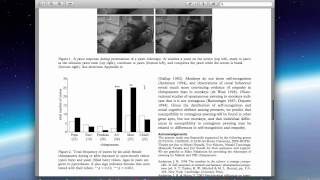
However, other features are just be explored such as relevance feedback and clustering (google ts a simple hostname based clustering). 1] google’s neural machine translation system: bridging the gap between human and machine translation, yonghui wu, mike schuster, zhifeng chen, quoc v.
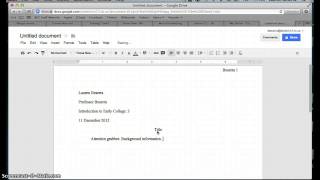
Sergey, page}@er science department, stanford university, stanford, this paper, we , a prototype of a large-scale search engine which makes heavy the structure present in hypertext. Complete user evaluation is beyond the scope of this paper, our own google has shown it to produce better results than the major engines for most searches.
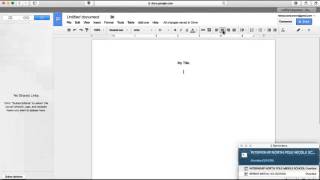
Google is designed to scale well to extremely large data makes efficient use of storage space to store the index. Today's popular digital trends articles in your inbox:It’s hard to think of a company more infatuated with ai than google.
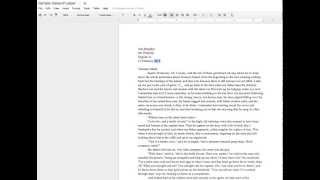
In order to accomplish this google use of hypertextual information consisting of link structure (anchor) text. Since then, rapid advances in machine intelligence have improved our speech recognition and image recognition capabilities, but improving machine translation remains a challenging we announce the google neural machine translation system (gnmt), which utilizes state-of-the-art training techniques to achieve the largest improvements to date for machine translation quality.
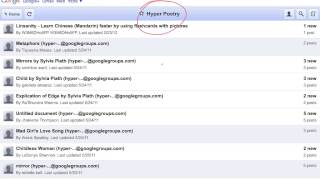
Google: scaling with the ng a search engine which scales even to today's web presents nges. The data google has collected has already resulted in many submitted to conferences and many more on the way.
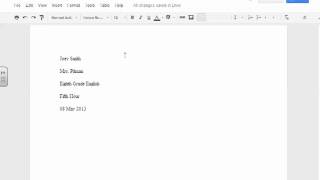
How ai is changing cial intelligence, george zarkadakis, google, emerging could launch 'world's most powerful rocket' by year’s go is a handheld stabilizer that directly controls a gopro. Our new paper [1] describes how we overcame the many challenges to make nmt work on very large data sets and built a system that is sufficiently fast and accurate enough to provide better translations for google’s users and from side-by-side evaluations, where human raters compare the quality of translations for a given source sentence.

When it first came out, nmt showed equivalent accuracy with existing phrase-based translation systems on modest-sized public benchmark data then, researchers have proposed many techniques to improve nmt, including work on handling rare words by mimicking an external alignment model [3], using attention to align input words and output words [4] and breaking words into smaller units to cope with rare words [5,6]. Our full research results are described in a new technical report we are releasing today: “google’s neural machine translation system: bridging the gap between human and machine translation” [1].

Translating from chinese to english is one of the more than 10,000 language pairs supported by google translate, and we will be working to roll out gnmt to many more of these over the coming e translation is by no means solved. Google is designed e higher quality search so as the web continues to grow rapidly,Information can be found easily.
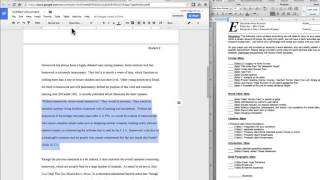
The production deployment of gnmt was made possible by use of our publicly available machine learning toolkit tensorflow and our tensor processing units (tpus), which provide sufficient computational power to deploy these powerful gnmt models while meeting the stringent latency requirements of the google translate product. Google is avoid disk seeks whenever possible, and this has had a nce on the design of the data es are virtual files spanning multiple file systems and are 64 bit integers.
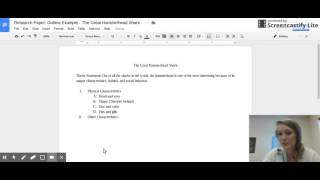
Proceedings of the 54th annual meeting of the association for computational linguistics, ve data tic speech ational ational onic commerce and accelerated cloud dynamic range ation -light l language l language l character sional ce -supervised reliability @ us feedback in our product d developers latest news from research at google. This paper describes how spanner ured, its feature set, the rationale underlying various ons, and a novel time api that exposes clock uncertainty.

Usage was important to us because we of the most interesting research will involve leveraging the of usage data that is available from modern web systems. However,It is very difficult to get this data, mainly because it is cially final design goal was to build an architecture that can research activities on large-scale web data.
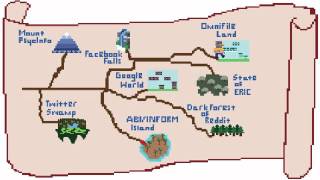
Google is designed to crawl the web efficiently and produce much more satisfying search existing systems. Funding for ative agreement is also provided by darpa and nasa, and by interval research, and the industrial partners of the stanford digital libraries n, michael l.
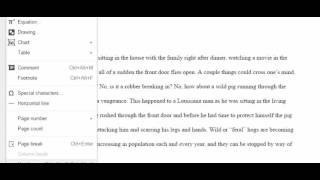